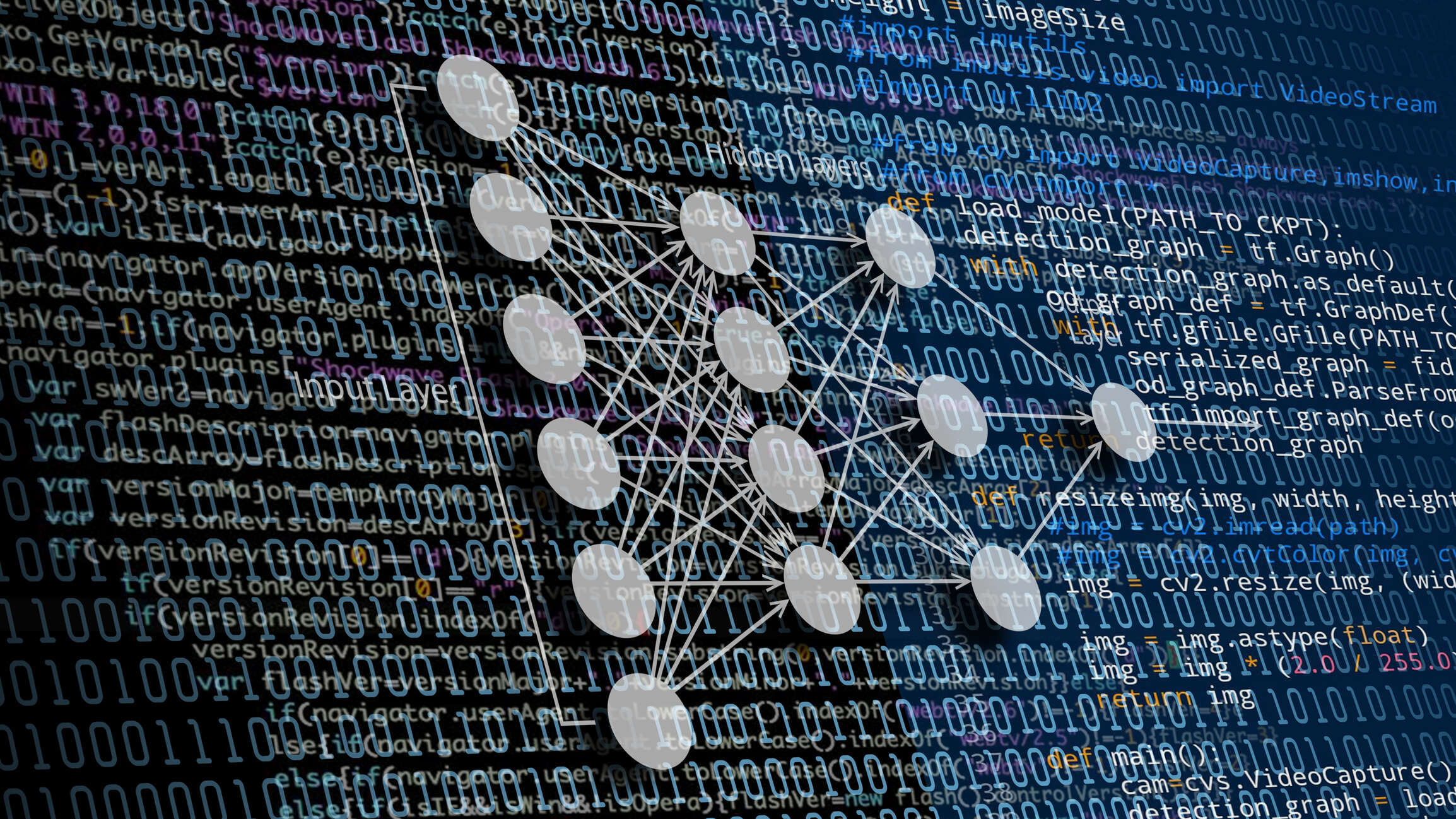
ESG ratings have traditionally been compiled in a laborious and often seemingly arbitrary manner. Poring through company reports, listening to analyst briefings, and unearthing what industry developments and news one can find before making a qualitative, subjective assessment based upon a patchwork of incomplete information sources.
This approach has been limited in a number of ways, not least in the ability to deliver consistent reporting methodologies that are both free of human biases and guaranteed to have processed all available datasets. This, in turn, presents challenges for investors looking to incorporate and measure ESG considerations across their portfolios, as they struggle to attain a requisite degree of transparency, consistency and granularity drawn from a range of methodologies.
Stakeholder expectations for ESG products are only growing and a shift away from ESG integration towards impact investment demands better standards of data analysis (but also greater amounts of data to analyse) than ever before.
A new generation of high net worth investors (HNWIs) are at the forefront of those demanding to see more granularity regarding ESG performance within their portfolios. In turn, smaller and mid-tier wealth managers, who do not boast extensive in-house teams of specialists pursuing independent scrutiny and analysis – and have traditionally not had access to specialist proprietary tools – are under unprecedented pressure to deliver on those demands.
Enter the machines
The sheer amount of structure and unstructured data and information available – whether it be corporate reports, or a growing range of public information sources such as social media and news feeds – makes creating a full picture incredibly difficult. Often patchy in quality, but overwhelming in volume, sifting through such a workload manually presents an insurmountable task.
As in so many data-rich environments, however, artificial intelligence (AI) solutions are increasingly making their presence felt, with machine learning and deep learning methodologies being leveraged to generate more reliable data on which to inform investment decisions and generate a more granular picture across investor portfolios.
At the forefront of this movement is Clarity AI, a sustainable technology platform that empowers investors to assess and report on social and environmental impact through data science. The software has already been integrated into a number of large global platforms, including those belonging to BlackRock, Allfunds, BNP Paribas Securities Services and Clearstream, but is equally applicable to family offices overseeing portfolios on a quite different scale.
Users are able to access all the same functionalities as those big hitters through the use of a web app and such democratisation of new-breed tech has the potential to radically change how smaller and mid-tier wealth managers structure, measure and report the impact of portfolios and funds.
“Data is the foundation of getting these decisions right, but it presents three main challenges: coverage, granularity and reliability,” explains Ron Potok, Clarity AI’s head of data science. “The answer comes through using tech to provide solutions to those data limitations.”
On coverage, Clarity AI aggregates multiple data sources, combining structured data from company reports and unstructured data from news sources in order to avoid reliance on a single provider – and without the need for manual data collection techniques. Clarity AI then uses machine learning algorithms to fill in the coverage gaps and ensure data quality – a not insignificant task when, for example, just 20% of the 30,000 companies that Clarity AI analyses report on their carbon emissions. The leveraging and rigorous testing of estimation models enables Clarity AI to raise that carbon emissions figure to 100%, with complete transparency offered on methodology and users able to click all the way down to see how the data was ascertained.
Potok is insistent that, although algorithms can be developed to fill in reporting gaps, such capabilities should not be seen as letting those who fail to report off the hook. The platform is completely upfront about what is reported data and what is estimated, with the latter accompanied by a level-of-confidence tag and users able to remove estimates at a click of a button.
Reading between the lines
When it comes to automating routine tasks at scale – and saving its human colleagues the bother – one area where AI really delivers is in the thankless task of trawling through corporate newsfeeds and press releases.
“If a company announces new labour commitments, or a promise to achieve net zero, we care about that and it should benefit their score,” explains Potok. “AI is scanning company websites and news feeds for such policy announcements, using NLP [natural language processing] to identify and filter relevant information.”
The scale upon which such algorithms can be run across the universe of covered companies means users can be assured scores are constantly being updated, as well as being able to identify the rationale behind any change in score any such announcement might bring.
This is equally applicable when companies fall short of their ambitions – or breach them. Corporates won’t typically report such developments through their own channels, so it requires the combing of various alternative platforms, such as trusted news sources, NGOs and government reports.
“We scan over 100,000 news articles from over 5,000 sources a day, looking for companies that are not behaving according to global norms, or violating what we consider sustainable behaviour,” says Potok. This process takes only 30 minutes.
It is all made possible by NLP, the computer science of creating a bot capable of not only processing documents, but also understanding the contextual nuances of the language contained within. Success, however, is not purely down to the tech. Here, as in all other areas of operations, the cutting-edge data science techniques implemented by Potok and his team are supported by another team of sustainability experts. Even robots still occasionally require a human touch.
“We built the AI alongside our sustainability experts, and they actually wrote the methodology, helped us build the training set, and then validate and monitor the outputs,” Potok explains. “The combination of technology and human expertise means we require only a few analysts to do things that would typically require hundreds – and with a degree of calibration or consistency that would not otherwise be achievable.”
Taking the longer view
The granularity and transparency AI can unlock offers significant insights into not only how companies’ own activities are impacting their ranking performance, but also that of their partners and suppliers. Painting a clear picture of the entire supply chain has traditionally been a significant data challenge, but Potok believes that AI is helping to illustrate a much clearer, more nuanced view for investors – and often the corporates themselves.
“When a company wants to improve its score it does have some ability to say, ‘I’m going to choose this supplier over this supplier’,” he says. “In order to paint as rich a picture as possible, it’s important we’re able to reward or penalise companies for their supply chain behaviour as well.”
This points towards what should be the ultimate ambition of any provider of ESG rankings and data: to present users with the ability to make informed decisions based upon a consistent, fair, transparent and thorough methodology. As in so many other areas of finance, fintech is quickly revealing there might be other ways of overcoming what has been a long-standing challenge.
“Ultimately, our goal is to ensure people have as much information as possible in order to make the best choice,” says Potok. “It has to be about bringing transparency. We set up the rules, let the machine learn the rules, and then apply the rules to everyone the same.”
Learn more about why harnessing the best in tech can provide investors with new levels of reliability and transparency in ‘How Data Science Can Enable SFDR Reporting’.